In the rapidly evolving landscape of data science and analytics, inferential statistics plays a pivotal role in predictive analytics. As we delve into 2024, understanding the integration of inferential statistics into predictive modeling unveils significant trends and innovations that are shaping the future of data-driven decision-making. This article explores how inferential statistics underpins predictive analytics, highlighting current trends and emerging innovations that are driving the field forward.
Understanding Inferential Statistics
Inferential statistics involves making inferences about a population based on a sample of data. Unlike descriptive statistics, which merely summarizes data, inferential statistics allows analysts to make predictions and draw conclusions that extend beyond the immediate data set. This capability is crucial in predictive analytics, where the goal is to forecast future trends and behaviors based on historical data.
The Intersection of Inferential Statistics and Predictive Analytics
Predictive analytics aims to use historical data to predict future outcomes. Inferential statistics provides the foundation for making these predictions by:
- Modeling Relationships: Inferential statistics helps in identifying and quantifying relationships between variables. Techniques such as regression analysis allow analysts to determine how changes in one variable are associated with changes in another, which is essential for building predictive models.
- Testing Hypotheses: Hypothesis testing is a core component of inferential statistics. By testing hypotheses about data, analysts can validate assumptions and refine predictive models. For example, if a company wants to predict customer churn, hypothesis testing can help determine which factors are statistically significant in influencing customer behavior.
- Estimating Parameters: Inferential statistics provides methods for estimating parameters of statistical models. Confidence intervals and point estimates derived from sample data help in understanding the precision and reliability of predictions.
Trends in Inferential Statistics for Predictive Analytics in 2024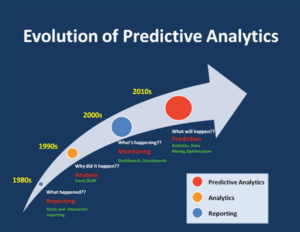
As we move through 2024, several key trends are emerging in the application of inferential statistics within predictive analytics:
- Integration with Machine Learning: The synergy between inferential statistics and machine learning is becoming more pronounced. Statistical techniques are increasingly being integrated into machine learning models to enhance their interpretability and robustness. For instance, statistical tests are used to validate the significance of features in machine learning algorithms.
- Advancements in Bayesian Statistics: Bayesian statistics, an approach that incorporates prior knowledge and updates predictions as new data becomes available, is gaining traction. This method allows for more nuanced predictions by continuously refining models with new evidence.
- Increased Use of Big Data: With the explosion of big data, inferential statistics is being applied to larger and more complex data sets. This trend necessitates the development of new statistical methods that can handle high-dimensional data while maintaining accuracy in predictions.
- Focus on Real-Time Analytics: Real-time data analysis is becoming crucial for industries like finance and healthcare. Inferential statistics is adapting to support real-time predictive modeling, enabling businesses to make immediate decisions based on current data.
- Ethical Considerations and Bias Mitigation: As predictive analytics impacts more aspects of daily life, there is a growing emphasis on ethical considerations. Inferential statistics is being employed to identify and mitigate biases in predictive models, ensuring that decisions are fair and equitable.
Innovations in Inferential Statistics for Predictive Analytics
Innovations in inferential statistics are continuously evolving, and several key developments are influencing predictive analytics:
- Enhanced Computational Techniques: Advances in computational power and algorithms are allowing for more sophisticated statistical modeling. Techniques such as Monte Carlo simulations and Markov Chain Monte Carlo (MCMC) are enabling more accurate and efficient predictions.
- Integration with Artificial Intelligence: The integration of inferential statistics with AI technologies is driving innovation. AI models are incorporating statistical methods to improve their accuracy and interpretability, leading to more reliable predictive analytics.
- Development of New Statistical Models: Researchers are developing new statistical models to address specific challenges in predictive analytics. For example, robust statistical models are being designed to handle noisy data and outliers more effectively.
- Interactive Data Visualization: Advances in data visualization tools are enhancing the ability to interpret statistical results. Interactive visualizations allow users to explore data and predictions more intuitively, facilitating better decision-making.
- Automated Statistical Analysis: Automation in statistical analysis is streamlining the process of building and evaluating predictive models. Tools that automate hypothesis testing, model selection, and parameter estimation are making inferential statistics more accessible and efficient.
Conclusion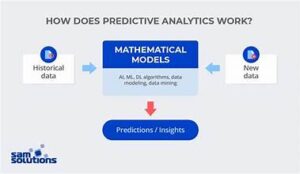
Inferential statistics remains a cornerstone of predictive analytics, providing the tools and methodologies needed to make informed predictions and decisions. As we progress through 2024, the integration of inferential statistics with emerging technologies and trends is driving innovation and enhancing the accuracy and applicability of predictive models. By staying abreast of these developments, analysts and data scientists can leverage inferential statistics to navigate the complexities of data and unlock valuable insights that drive business success and societal progress.