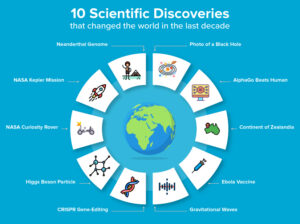
In 2024, probability theory continues to be at the heart of data science advancements. The rapid pace of technological progress and the increasing complexity of data require new approaches to probability that drive innovation and enhance the power of data science. This article delves into the latest breakthroughs in probability theory and explores how they are revolutionizing the field of data science.
1. Quantum Probability Theory
One of the most exciting developments in probability theory is the integration of quantum mechanics into probabilistic models. Quantum probability theory extends classical probability concepts to account for quantum phenomena, providing a new framework for understanding complex data relationships. This approach is particularly valuable in fields like cryptography, quantum computing, and advanced machine learning algorithms.
Quantum probability offers several advantages:
- Enhanced Data Analysis: Quantum models can process and analyze data in ways that classical models cannot, leading to more accurate predictions and insights.
- Increased Efficiency: Quantum algorithms can potentially solve complex problems faster than traditional algorithms, reducing computational time and resources.
2. Bayesian Deep Learning
Bayesian deep learning combines Bayesian probability with deep learning techniques to improve model performance and reliability. By incorporating Bayesian methods, this approach allows for the quantification of uncertainty in predictions, which is crucial for making robust decisions in uncertain environments.
Key benefits of Bayesian deep learning include:
- Uncertainty Estimation: Bayesian methods provide a measure of confidence in predictions, which helps in assessing the reliability of the model.
- Improved Generalization: Bayesian deep learning models are less likely to overfit the training data, leading to better performance on unseen data.
3. Advances in Copula Theory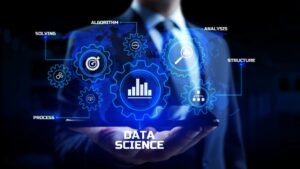
Copula theory, which deals with the dependencies between random variables, has seen significant advancements. Modern copula methods allow for a more flexible modeling of complex dependencies in multivariate data. These advancements are crucial for applications such as finance, risk management, and insurance.
Recent developments in copula theory include:
- Flexible Dependence Structures: New copula models offer improved flexibility in capturing various types of dependencies between variables.
- Enhanced Estimation Techniques: Advances in estimation methods provide more accurate and efficient ways to fit copula models to data.
4. Nonparametric Probability Models
Nonparametric probability models do not assume a fixed form for the underlying probability distribution, allowing for greater flexibility in modeling complex data. These models are particularly useful in scenarios where the data does not fit traditional parametric models.
Notable advancements in nonparametric probability models include:
- Dirichlet Process Mixtures: These models allow for an infinite mixture of components, which is useful for clustering and density estimation tasks.
- Gaussian Processes: Gaussian processes offer a powerful framework for regression and classification tasks by providing a nonparametric approach to modeling functions.
5. Integration of Machine Learning and Probability Theory
The intersection of machine learning and probability theory has led to significant innovations in how data is processed and analyzed. Probabilistic machine learning models leverage probability theory to improve learning algorithms and make more informed predictions.
Key developments in this area include:
- Probabilistic Graphical Models: These models use graph theory to represent and analyze complex probabilistic relationships between variables.
- Reinforcement Learning: Advances in reinforcement learning algorithms incorporate probabilistic methods to optimize decision-making processes in dynamic environments.
6. Practical Applications and Implications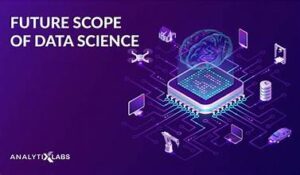
The breakthroughs in probability theory are having a profound impact on various industries:
- Finance: Enhanced risk modeling and forecasting techniques help in managing financial risks and optimizing investment strategies.
- Healthcare: Improved data analysis methods lead to better patient outcomes and more personalized treatments.
- Marketing: Advanced probabilistic models enable more effective targeting and personalization of marketing strategies.
Conclusion
The latest breakthroughs in probability theory are transforming the landscape of data science, offering new tools and techniques for handling complex data challenges. From quantum probability to advancements in nonparametric models, these innovations are driving progress across various fields and industries. As data science continues to evolve, the integration of cutting-edge probability theory will undoubtedly play a crucial role in shaping the future of data analysis and decision-making.