
In the rapidly evolving world of technology, machine learning (ML) algorithms are at the forefront of innovation, driving transformative changes across various sectors. From enhancing data analytics to powering intelligent systems, these algorithms are not just shaping the future—they are actively creating it. This article explores how cutting-edge machine learning algorithms are influencing the technological landscape, providing insights into their impact and future potential.
1. Revolutionizing Data Analysis
Machine learning algorithms have revolutionized data analysis by enabling systems to learn from and interpret vast amounts of data with unprecedented accuracy. Traditional data analysis methods often struggled with the sheer volume and complexity of modern datasets. ML algorithms, particularly those based on deep learning and neural networks, have significantly improved the ability to extract meaningful insights from data.
Example: In healthcare, ML algorithms analyze patient records to identify patterns and predict disease outbreaks, leading to more accurate diagnoses and personalized treatment plans. This capability enhances decision-making and operational efficiency in medical settings.
2. Driving Innovations in Artificial Intelligence
The field of artificial intelligence (AI) is closely intertwined with machine learning. Advanced ML algorithms contribute to the development of sophisticated AI systems capable of performing complex tasks. These include natural language processing (NLP), computer vision, and autonomous systems.
Example: Self-driving cars utilize machine learning algorithms to process real-time data from sensors and cameras, making instantaneous decisions to navigate roads safely. This technology promises to transform transportation by reducing human error and increasing road safety.
3. Enhancing Personalization and User Experience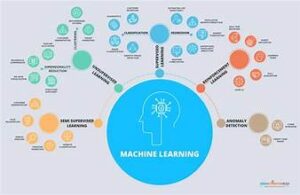
One of the most noticeable impacts of machine learning is the enhancement of personalization across digital platforms. ML algorithms analyze user behavior and preferences to deliver tailored experiences, recommendations, and content.
Example: Streaming services like Netflix and Spotify use machine learning to analyze viewing and listening habits, providing personalized recommendations that enhance user satisfaction and engagement. This level of customization keeps users engaged and helps businesses retain customers.
4. Optimizing Business Operations
Machine learning algorithms play a crucial role in optimizing business operations by improving efficiency, reducing costs, and enhancing decision-making processes. They enable predictive maintenance, demand forecasting, and fraud detection, among other applications.
Example: In the retail industry, ML algorithms predict inventory needs based on sales trends and seasonal patterns. This predictive capability helps businesses manage stock levels efficiently, minimizing both overstock and stockouts.
5. Transforming Cybersecurity
As cyber threats become increasingly sophisticated, machine learning algorithms offer advanced solutions for enhancing cybersecurity. ML models can detect anomalies, identify potential threats, and respond to cyber attacks in real-time.
Example: Security systems powered by machine learning analyze network traffic patterns to identify unusual activities that may indicate a security breach. This proactive approach helps in mitigating potential threats before they escalate.
6. Facilitating Smart Cities Development
Machine learning algorithms contribute to the development of smart cities by optimizing various urban systems, including traffic management, energy consumption, and public safety. These algorithms analyze data from sensors and other sources to make cities more efficient and livable.
Example: Traffic management systems in smart cities use ML algorithms to analyze real-time traffic data, adjust traffic signals, and optimize routes to reduce congestion and improve traffic flow.
7. Advancing Scientific Research
Machine learning is accelerating scientific research by enabling researchers to process and analyze large datasets more efficiently. This capability is particularly valuable in fields such as genomics, climate science, and materials science.
Example: In genomics, ML algorithms analyze genetic data to identify correlations between genetic variations and diseases. This information accelerates the development of new treatments and personalized medicine.
8. Ethical Considerations and Future Challenges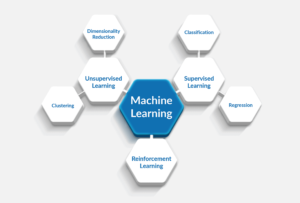
As machine learning algorithms become more integrated into various aspects of life, ethical considerations and challenges emerge. Issues such as data privacy, algorithmic bias, and transparency are critical areas that need addressing to ensure the responsible use of ML technologies.
Example: Ensuring that ML algorithms are free from bias and provide fair outcomes is crucial for maintaining trust and fairness in systems that impact people’s lives, such as hiring practices and loan approvals.
Conclusion
Cutting-edge machine learning algorithms are shaping the future of technology by transforming data analysis, advancing AI, enhancing personalization, optimizing business operations, and improving cybersecurity. As these technologies continue to evolve, they hold the potential to drive further innovations and address complex challenges across various domains. However, it is essential to navigate the ethical considerations and challenges associated with ML to ensure that its benefits are realized in a responsible and equitable manner.