In 2024, the landscape of machine learning (ML) continues to evolve at a breakneck pace, driving innovation across various industries. From healthcare to finance, these cutting-edge algorithms are reshaping how businesses operate, making them more efficient, predictive, and customer-focused. This article delves into some of the most impactful machine learning algorithms of the year, exploring their applications and how they are revolutionizing different sectors.
1. Transformers and Their Variants
Transformers, initially designed for natural language processing, have expanded their influence across diverse fields. The core architecture, introduced in 2017, has given rise to numerous variants, such as GPT-4 and BERT, which excel in understanding context and generating human-like text. In 2024, transformers are not only enhancing conversational AI but are also applied in fields like computer vision and genomics.
Applications:
- Healthcare: Transformers are being used to analyze medical literature and assist in diagnostics by predicting patient outcomes based on historical data.
- Finance: They are deployed in fraud detection and financial forecasting, offering improved accuracy by interpreting complex data patterns.
2. Reinforcement Learning (RL)
Reinforcement Learning has made significant strides, particularly in optimizing decision-making processes. In 2024, RL algorithms are being used to fine-tune complex systems by learning from interactions with their environment and making decisions that maximize long-term rewards.
Applications:
- Robotics: RL is used to enhance robot performance in dynamic environments, such as manufacturing lines and autonomous vehicles.
- Supply Chain Management: It helps in optimizing inventory management and logistics by predicting demand and adjusting operations in real-time.
3. Federated Learning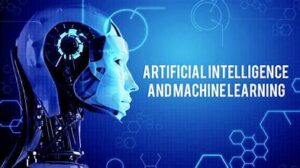
Federated Learning addresses the challenge of data privacy by enabling models to be trained across decentralized devices without transferring raw data. This year, it has gained traction due to increasing concerns about data security and privacy.
Applications:
- Healthcare: Federated Learning allows hospitals to collaborate on research and improve diagnostics without sharing sensitive patient data.
- Smart Devices: It enhances user experiences by allowing devices to learn from local data while ensuring user privacy.
4. Graph Neural Networks (GNNs)
Graph Neural Networks are designed to handle graph-structured data, which is crucial for understanding relationships and dependencies between entities. In 2024, GNNs are making waves by improving performance in tasks where data can be represented as networks or graphs.
Applications:
- Social Networks: GNNs are used to predict user behavior and recommend connections based on interactions and relationships within the network.
- Drug Discovery: They help in identifying potential drug interactions and predicting molecular properties by analyzing chemical compounds’ graph structures.
5. AutoML
Automated Machine Learning (AutoML) is democratizing access to advanced ML techniques by automating the process of model selection, training, and optimization. This year, AutoML tools are becoming more accessible, allowing non-experts to build high-performing models with minimal effort.
Applications:
- Retail: AutoML is used to develop personalized marketing strategies and optimize product recommendations.
- Real Estate: It assists in predicting property values and market trends, providing insights for investment decisions.
6. Generative Adversarial Networks (GANs)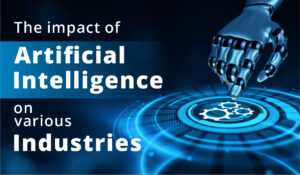
Generative Adversarial Networks, known for their ability to generate realistic synthetic data, continue to advance in 2024. GANs are increasingly used in creative fields and beyond, pushing the boundaries of what AI can create.
Applications:
- Entertainment: GANs are used to create realistic animations and visual effects, enhancing the production quality of films and video games.
- Fashion: They assist in designing new clothing patterns and styles by generating novel fashion designs.
7. Self-Supervised Learning
Self-Supervised Learning is an emerging paradigm that leverages unlabeled data to train models. This approach has gained momentum as it reduces the need for labeled datasets, which can be expensive and time-consuming to create.
Applications:
- Text Analysis: It is used to understand and generate human-like text by learning from vast amounts of unstructured text data.
- Image Processing: Self-Supervised Learning improves object recognition and image segmentation by utilizing large-scale image datasets.
Conclusion
The machine learning algorithms of 2024 are transforming industries by enhancing capabilities, improving efficiency, and driving innovation. From transformers and reinforcement learning to federated learning and GANs, these technologies are not only solving complex problems but also opening new avenues for growth and development. As these algorithms continue to evolve, their impact on various sectors will likely expand, paving the way for a smarter and more interconnected world.